Background: population screening for lung cancer using LDCT and AI
Screening means testing for a disease when there are no symptoms or (familial) history of that disease, and involves tests that aim to detect early signs of a disease, genetic predispositions or risk factors for a specific disease. Population screening refers to screening tests that are offered to all individuals in a target group, usually defined by age, as part of an organized program. It is a public health service in which members of a population at risk for a disease or its complications are asked questions or offered a test, to identify those individuals who are at sufficiently high risk that further diagnostic tests or treatments are justified (source: www.htaglossary.net). The most well-known are probably population screening programs offered by the government, like for example population screening for breast cancer and the heel prick test for newborn babies. A population screening program must include all the core components in the screening process from inviting the target population to offering effective treatment for individuals diagnosed with a disease.
Lung cancer is one of the most common types of cancer, leading to the highest amount of cancer-related deaths, worldwide.1 At present, the majority of lung cancer cases are diagnosed in advanced stages, without screening, resulting in significant treatment-related costs with little or no survival benefits.2
The recommended screening test for lung cancer is low-dose computed tomography (also called a low-dose CT scan, or LDCT). During an LDCT scan, a person lies on a table and an X-ray machine uses a low dose of radiation to make detailed images of the lungs. The scan only takes a few minutes and is not painful. Collectively, these observations seem to make a strong case for improving early diagnosis and introducing lung cancer population screening.
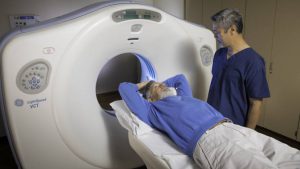
But despite the high burden of disease, and the relevance of early diagnosis, there are some controversies related to population screening for lung cancer 2:
–> From a radiologist’s perspective, an important issue is the anticipated increase in the number of large-volume imaging data sets that need to be evaluated, leading to an extensive increase of workload. In addition, there are issues with imaging protocols, reliable and reproducible nodule measurement tools (i.e. nodules are small masses of tissue in the lung that appear as round, white shadows on a chest CT scan; larger lung nodules can be malignant, i.e. cancer). Technological innovations such as standardization of LDCT protocols and improved computer-aided detection (CAD) software, using artificial intelligence, could address these issues but also demand a shift to other routines.
–> Given the rapid rate of developments in artificial intelligence techniques for analyzing medical images, especially the approach of deep learning (i.e. a set of techniques and algorithms that enable computers to disover complicated patterns in large data sets, see the background information below for an explanation) it is also difficult to forecast how this will influence the performance of a potential screening program. The use of artificial intelligence in healthcare also raises questions related to responsibilities (e.g. who is responsible when a computer algorithm misinterprets a LDCT scan?) and our abilities to comprehend the test results (e.g. can we explain how a deep learning algorithm has analyzed a LDCT scan?).
–> Because LDCT scans have to be made annually, there is some discussion on the acceptable radiation dose and the potential risk for radiation-induced cancer.
–> LDCT screening has been associated with overdiagnosis; false positives; patients with benign conditions undergoing minor and major invasive follow-up procedures.3
–> The introduction of a population screening program requires the allocation of healthcare resources (time, expertise, money) that can not be spent on other interventions, and asks from society (including non-smokers) to cover the costs for a disease that is (primarily) self-inflicted by smoking.
To conclude, the potential introduction of a lung cancer screening program poses a challenge to policy decision makers that is related to its feasibility and desirability.
Background: Deep learning
Machine learning, the scientific study of algorithms and statistical models that computer systems use to perform a specific task without using explicit instructions, has seen some dramatic developments recently. These are driven by breakthroughs in artificial neural networks, often termed deep learning, a set of techniques and algorithms that enable computers to discover complicated patterns in large data sets. Feeding these breakthroughs is the increased access to, and collection of, data (“big data”), user-friendly software and an explosion of the available computing power; which enables the use of neural networks that are deeper than ever before.4 Deep learning obtained its prominent position in computer vision (the scientific field that deals with how computers can gain high-level understanding of digital images or videos) when neural networks started outperforming other available methods.
Healthcare providers generate and store enormous amounts of data which could potentially contain valuable information, at a pace that surpasses traditional methods of data analysis. Machine learning therefore enters the picture, because it is one of the best ways of integrating, analysing and making predictions based on large and various data sets. Applications in healthcare range from the analysis of biomedical data, to predicting medical events, to computer-aided detection, diagnosis and supporting clinicial decision making.
Deep learning can be used in radiology practices for efficiency improvement, image processing and the automatic analysis of images to detect and diagnose disease. Deep learning is well suited to medical big data, and can be used to extract useful knowledge from it.5 It is expected to help radiologists with providing more accurate diagnosis, by delivering quantitative analysis of images and enabling a shorter time needed to interpret all these images.
If you want to know something about how deep learning works, you could watch this video which explains the idea in 5 minutes:
Notes
- https://www.who.int/news-room/fact-sheets/detail/cancer
- Gill, R. R., Jaklitsch, M. T., & Jacobson, F. L. (2013). Controversies in lung cancer screening. Journal of the American College of Radiology, 10(12), 931-936.
- Ali, M. U., Miller, J., Peirson, L., Fitzpatrick-Lewis, D., Kenny, M., Sherifali, D., & Raina, P. (2016). Screening for lung cancer: a systematic review and meta-analysis. Preventive medicine, 89, 301-314.
- Lundervold, A. S., & Lundervold, A. (2019). An overview of deep learning in medical imaging focusing on MRI. Zeitschrift für Medizinische Physik, 29(2), 102-127.
- Lee, J. G., Jun, S., Cho, Y. W., Lee, H., Kim, G. B., Seo, J. B., & Kim, N. (2017). Deep Learning in Medical Imaging: General Overview. Korean journal of radiology, 18(4), 570–584.